Phases of Artificial Intelligence
Artificial Intelligence (AI) has grown from a basic idea into one of the most powerful technologies in the world. Its journey has gone through several major phases, each building on the last. Understanding these phases helps us see how AI started, where it is now, and where it’s going in the future. Let’s explore the important stages that define the history and development of AI.
1. Theoretical Phase
Long before computers existed, people dreamed of intelligent machines. This phase includes early ideas, myths, and theories about artificial beings that could think and act like humans. Writers and philosophers imagined mechanical men and thinking machines. These ideas were just imagination, but they laid the foundation for the real progress to come later.
Ancient Myths and Dreams
Stories from ancient Greece, China, and other cultures spoke of artificial life. In Greek mythology, Hephaestus built golden robots. In China, inventors created moving dolls. These myths showed a deep human interest in creating intelligence outside the human body.
Philosophical Questions
Philosophers like Descartes and Leibniz asked questions about the mind, logic, and machines. Could thinking be explained by rules? Could machines follow these rules and think? These early thoughts shaped the later ideas of computer science and AI.
2. Rule-Based AI (Symbolic AI)
In the 1950s, AI became a real scientific field. This phase is known as Symbolic AI or Rule-Based AI. Scientists tried to make machines intelligent by giving them rules and logic. These rules helped the machines "think" in a human-like way, but only in limited areas.
Birth of AI
In 1956, a workshop at Dartmouth College officially started the AI field. John McCarthy, Marvin Minsky, and other researchers believed machines could be made to think like humans. They built simple programs that solved puzzles and played games.
Expert Systems
In the 1970s and 80s, expert systems became popular. These programs used "if-then" rules to mimic the decision-making of human experts. For example, an expert system in medicine could help diagnose diseases. However, these systems were hard to build and couldn’t learn new things.
Limitations
Rule-based AI could only do what it was told. It couldn’t understand the world or improve by itself. As problems became more complex, these systems struggled. This led to periods of slow progress, often called AI winters.
3. Machine Learning Phase
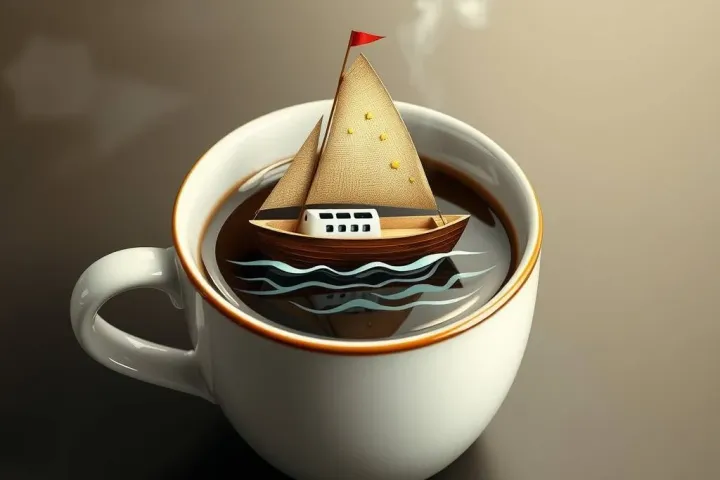
In the 1990s, AI entered a new phase called Machine Learning (ML). Instead of writing rules, scientists began teaching machines to learn from data. This made AI much more flexible and powerful. Machines could now find patterns, make predictions, and improve over time.
Learning from Data
Machine learning allows computers to learn without being directly programmed. Algorithms are trained on large datasets and learn to make decisions based on examples. For example, a spam filter can learn what spam looks like by studying thousands of emails.
Key Algorithms
Some of the most common machine learning algorithms include decision trees, support vector machines, and clustering methods. These tools became the core of many modern AI applications.
Growth of Applications
During this phase, AI started appearing in more places. It powered search engines, product recommendations, fraud detection, and even simple voice recognition. But it was still limited in how deeply it understood language or images.
4. Deep Learning Phase
The next big step was Deep Learning. Starting in the early 2010s, deep learning changed everything. This phase uses artificial neural networks with many layers, inspired by the human brain. These networks can handle huge amounts of data and perform very complex tasks.
Neural Networks
A neural network is made of nodes (like neurons) that are connected in layers. Data passes through these layers, and the network learns by adjusting weights on the connections. With many layers, these networks can learn very deep patterns.
Breakthroughs
Deep learning led to major breakthroughs in speech recognition, image analysis, and natural language understanding. AI systems became better than humans in some areas, like recognizing images or playing video games.
Famous Examples
Google's DeepMind created AlphaGo, which beat the world champion in the game of Go. Self-driving cars became a reality, powered by deep learning. Voice assistants like Siri, Alexa, and Google Assistant also rely on deep learning to understand and respond.
5. Cognitive AI Phase
In this phase, AI goes beyond pattern recognition. Cognitive AI tries to simulate human thought processes. It includes understanding context, emotions, reasoning, and even memory. The goal is to make machines think more like humans.
Natural Language Understanding
Modern AI models like GPT (Generative Pre-trained Transformers) can understand and generate human-like text. They can write essays, answer questions, and even chat in a natural way. These systems try to grasp not just the words, but the meaning behind them.
Emotion and Context
Cognitive AI also tries to detect emotions from voice or text and respond accordingly. In customer service, for example, AI can tell if a user is frustrated and change its tone or offer extra help.
Memory and Reasoning
Some AI systems now use memory to recall past interactions and reason about new situations. This brings machines one step closer to human thinking. It also helps AI act more consistently over time.
6. General AI Phase (Still in Progress)
General AI (also called AGI or Artificial General Intelligence) is the dream of creating machines that can do anything a human can do. Unlike narrow AI, which focuses on one task, AGI would be flexible and adaptable. It could learn new skills, solve different types of problems, and think creatively.
Why It’s Hard
Building general intelligence is extremely difficult. It requires understanding not just data, but the real world, emotions, ethics, and logic. AGI would need to reason across many fields and apply knowledge like a human.
Current Research
Researchers are making slow progress toward AGI. Large language models, brain-inspired computing, and new training methods are pushing the boundaries. But experts believe we are still years—or decades—away from true AGI.
Potential Risks and Benefits
AGI could solve major problems like climate change, disease, and education. But it could also pose risks if not controlled properly. Many scientists are calling for careful planning and ethics in AGI development.
7. Superintelligent AI (Future Possibility)
Superintelligent AI refers to machines that are much smarter than humans in every way. This phase hasn’t happened yet, but it is a popular topic in science fiction and long-term planning. A superintelligent AI could make decisions faster, learn quicker, and solve problems that are too complex for humans.
The Debate
Some thinkers like Elon Musk and Stephen Hawking warned about the dangers of superintelligence. They say we must build safety rules now. Others believe it could be the key to a better future if developed responsibly.
AI Safety and Control
To prepare for superintelligent AI, researchers are exploring ways to align AI with human values. They’re working on making AI explain its actions and follow ethical guidelines. The goal is to keep humans in control at all times.
Conclusion
Artificial Intelligence has moved through many phases, from simple ideas to powerful deep learning systems. Each phase brought us closer to machines that can think, learn, and even interact like humans. Today, AI helps us in many areas—healthcare, business, education, and entertainment. The future may bring even more exciting and challenging changes. By understanding these phases, we can better guide the path of AI to be safe, fair, and helpful for everyone.